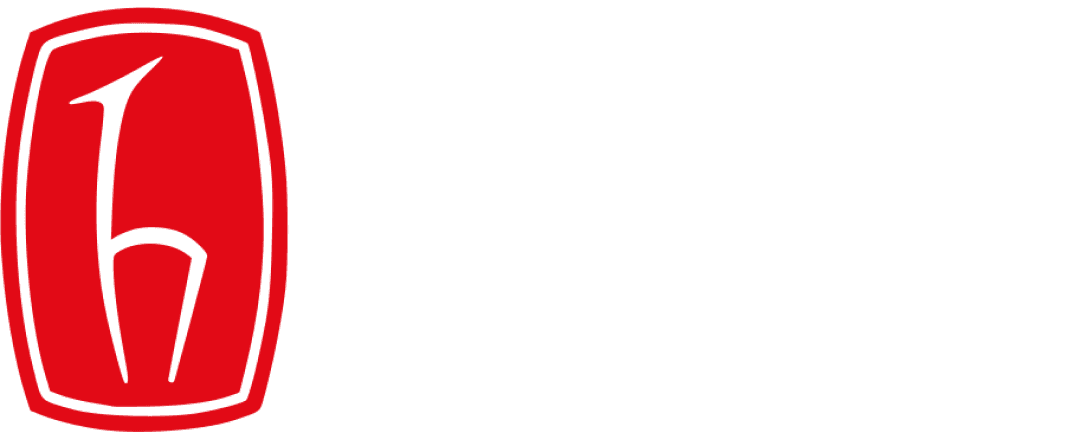
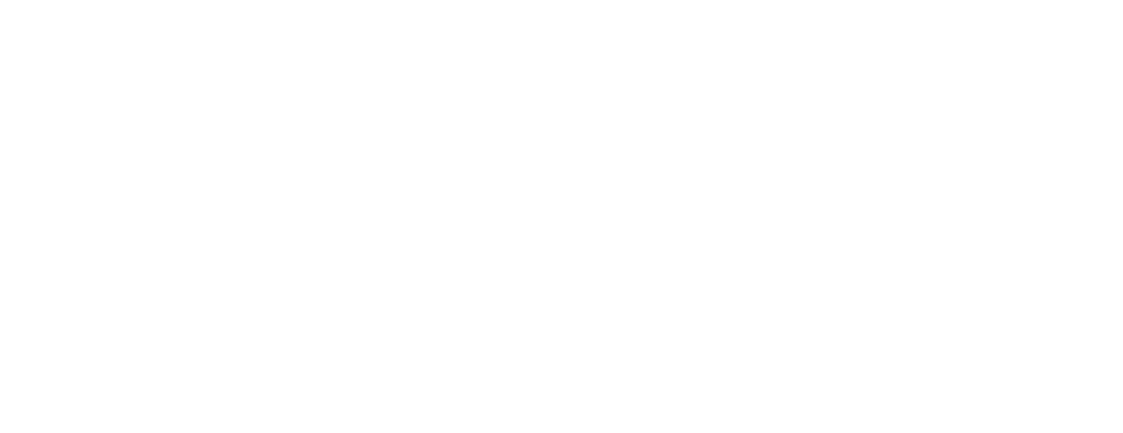
Vision Beyond Sight
Introduction
Today, over 285 million people worldwide live with visual impairments—39 million of them are completely blind World Health Organization.While assistive technology has come a long way, traditional tools often fall short in adapting to real-world environments. That's where AI and Vision-Language Models (VLMs) step in—offering the ability to understand both images and language for smarter, more responsive assistance.At Beyond Vision, we're building modular, AI-powered systems that help visually impaired individuals navigate safely, interact independently, and access information in real time—bringing us closer to a more inclusive and accessible world.
Methodology
Dataset
Curated comprehensive dataset of urban environments, ATMs, obstacles, and navigation scenarios. Includes diverse lighting conditions, weather situations, and real-world scenarios to ensure robust model training and validation.
YOLO Detection
Specialized YOLOv8 models trained for ATM detection and obstacle recognition. Features real-time object detection, segmentation, and tracking capabilities optimized for low-latency mobile deployment.
Advanced Algorithms
Integration of EasyOCR for text recognition, sophisticated distance calculation, and danger assessment algorithms. Real-time processing for immediate hazard detection and safety alerts.
Mobile Application
User-friendly mobile interface with advanced voice interaction capabilities. Features intuitive navigation controls, real-time feedback, and customizable accessibility settings for enhanced user experience.
Vision-Language Models
Integration of LLaVA and GPT models for advanced scene understanding and natural language interaction. Enables detailed scene descriptions and contextual assistance for users.
Cloud Infrastructure
Scalable cloud architecture for model deployment and real-time processing. Ensures high availability, low latency, and seamless updates while maintaining data security and privacy.
Core Modules
Attention
Apply
Communicate
Key Results & Achievements
Object Detection Excellence
Real-time Processing Power
Advanced Text Recognition
Intelligent Visual Understanding
Our system achieved remarkable accuracy with 91.3% in campus obstacle detection and 89.4% for ATM interface elements, setting new standards in assistive technology.
Analysis
Overall Performance
Object Class Detection Accuracy
Conclusion
Impact & Innovation
Our project represents a transformative approach to how visually impaired individuals interact with their environment, particularly focusing on two critical areas: ATM usage and campus navigation. By enabling independent access to these essential services, we're taking significant steps toward greater autonomy.
Key Features
ATM Module
Features finger-tracking technology with real-time spoken feedback, powered by YOLOv8 for precise finger and button detection, complemented by EasyOCR for accurate text recognition.
Navigation Module
Utilizes a custom-trained YOLOv8 model on thousands of campus images to identify obstacles and provide timely safety alerts, enhancing independent mobility.
Future Directions
- Enhanced model accuracy through expanded dataset collection
- Implementation of lightweight AI models for mobile devices and smart glasses
- Integration of user feedback for improved accessibility
- Real-world testing in banking and educational environments
While currently in its conceptual phase, this project demonstrates significant potential for real-world impact. With continued development and support, it could evolve into a powerful tool that meaningfully enhances the independence and quality of life for visually impaired individuals.
References
Research & Statistics
- WHO (2014)Visual impairment and blindness: Fact Sheet N°282
- Zhang, Q., et al. (2019)Smartphone-based navigation aid using camera and GPS
- Kumar, A., et al. (2020)Wearable obstacle detection system
Implementation Studies
- Johnson, M., et al. (2021)Computer vision-based ATM access system
- Park, H., et al. (2020)Text recognition on ATM screens using OCR
- Wang, C., et al. (2022)Domain-specific fine-tuning of YOLO